Master the One-Sample T-Test Formula
Staff Writer • April 1, 2025 • Analytics, Marketing
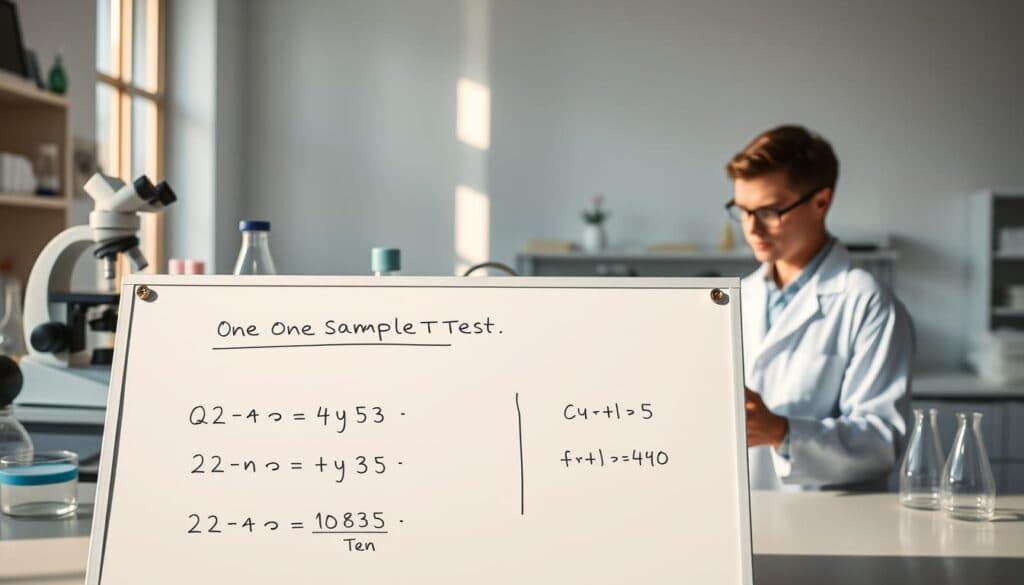
95% of scientific research uses statistical hypothesis testing to validate discoveries. The one-sample t-test formula is crucial for this analysis. It lets researchers compare sample means to theoretical benchmarks accurately.
Statistical hypothesis testing turns raw data into meaningful insights. The one-sample t-test formula helps evaluate if a sample’s mean differs from a set population mean. It’s a powerful tool for professionals in various fields.
This method isn’t just for academics. It’s a vital skill for business analysts and decision-makers. Mastering the one-sample t-test allows you to make confident, evidence-based decisions.
Key Takeaways
- One-sample t-test evaluates differences between sample and theoretical means
- Statistical hypothesis testing requires normally distributed data
- The t-test formula helps determine statistical significance
- Applicable across research, business, and scientific domains
- Provides objective evidence for decision-making processes
Understanding the Fundamentals of One-Sample T-Tests
The one-sample t-test is a powerful tool for data analysis. It helps determine if a sample mean differs from a population mean. This method, created in 1908, offers valuable insights from data. 🧠📊
What is a One-Sample T-Test?
A one-sample t-test compares a sample mean to a known population mean. It works best for small samples, usually less than 30 observations. The test reveals if differences are statistically significant or random. 🔍
When to Use a One-Sample T-Test
- Comparing a sample mean to a known population mean
- Analyzing experimental results with small sample sizes
- Determining statistical significance in research studies
- Evaluating process improvements in business and manufacturing
Key Components of Statistical Testing
The null hypothesis suggests no significant difference exists. The alternative hypothesis proposes a statistically significant difference. The significance level, often 0.05, sets the threshold for rejecting the null hypothesis. 📈
One-sample t-tests require several key assumptions:
- Data must be continuous or ordinal
- Observations should be randomly selected
- Data should approximate a normal distribution
- Sample size should be appropriate for analysis
Understanding these concepts helps you conduct robust statistical analyses. You’ll make better data-driven decisions in research and business. 🚀
One-Sample T-Test Formula and Its Components
The one-sample t-test is a key tool in statistical analysis. It compares a sample mean to a known population mean. This test provides vital insights across many fields. 🔬
Breaking Down the Formula Elements
The one-sample t-test formula is t = (M – μ) / (σ / √n). Let’s explore its components:
- M: Sample mean
- μ: Hypothesized population mean
- σ: Sample standard deviation
- n: Sample size
Understanding Degrees of Freedom
Degrees of freedom are crucial in t-distribution calculations. They’re calculated as n – 1. This value shapes the t-distribution curve and affects statistical significance. 📊
Component | Description | Calculation Method |
---|---|---|
Degrees of Freedom | Number of independent values | Sample Size – 1 |
Sample Mean | Average of sample data points | (Sum of all values) / (Number of values) |
Standard Deviation | Measure of data variability | Square root of variance |
Calculating Test Statistics
The significance level is vital in a one-sample t-test. It’s usually set at 0.05. This threshold determines if results are statistically meaningful.
The t-distribution provides a framework for interpreting test statistics. 🧮
Each formula component plays a role in the overall statistical analysis. Practice and careful calculation will improve your skills. Master this essential technique! 👩🔬🔍
Setting Up Statistical Hypotheses and Assumptions
Statistical hypothesis testing starts with clear, precise hypotheses. For a one-sample t-test, researchers must define null and alternative hypotheses carefully. This ensures accurate analysis. 🎯
Hypothesis testing involves one-tailed and two-tailed tests. These help evaluate if a sample mean differs from a population mean. A one-tailed test checks if the sample mean is greater or less than a value.
A two-tailed test looks for any significant difference in either direction. Both approaches are crucial for scientific evaluation.
Key assumptions for a valid one-sample t-test include:
- Data must be randomly sampled
- Measurements should be metric in scale
- Data distribution should approximate normality
- No significant outliers present in the dataset
Researchers follow a structured approach when setting up hypotheses. The null hypothesis (H₀) assumes no significant difference from the expected value. The alternative hypothesis (Hₐ) suggests a meaningful statistical difference.
This framework allows for precise evaluation of research questions across various fields. It helps researchers draw meaningful conclusions from their data. 🧐
Understanding these principles ensures robust statistical analysis. Careful hypothesis formulation is key to reliable scientific insights. 🚀
Step-by-Step Guide to Performing the Test
Statistical hypothesis testing needs a careful approach for precise analysis. A structured method helps researchers confidently navigate the one-sample t-test process. Let’s explore the key steps. 🔍
Data Collection and Preparation
Proper data preparation is crucial for effective statistical hypothesis testing. It forms the foundation for reliable results.
- Collect a sample size of under 30 observations for reliable population insights
- Verify data normality using statistical software
- Check for potential outliers that might skew results
- Ensure data independence between observations
Calculating Test Statistics
Test statistics calculation involves several important steps. These components are essential for accurate results.
- Compute the sample mean (x̄)
- Determine the population mean (μ)
- Calculate standard deviation
- Apply the t-test formula: t = (x̄ – μ) / (σ / √n)
Interpreting P-Values
P-values play a vital role in determining statistical significance. The standard confidence level is 0.05.
- P-value
- P-value > 0.05: No significant difference detected
Professional tip: Use statistical software like Python’s SciPy library for complex calculations. It ensures accuracy in your hypothesis testing. 💻
Statistical Significance and Effect Size
Statistical significance and effect size are key in understanding research results. Researchers use these tools in one-sample t-tests to examine their findings. They help determine if results are meaningful and important. 🔍
The significance level is usually 0.05. It shows if results are statistically meaningful. If the p-value is lower, you can reject the null hypothesis.
Key Concepts in Statistical Analysis
- Significance Level: Determines the threshold for statistical significance
- P-Value: Indicates the probability of obtaining results by chance
- Effect Size: Measures the practical importance of your findings
Cohen’s d is the main measure of effect size for t-tests. It helps researchers understand the importance of differences beyond statistics. 🧐
Effect Size | Cohen’s d Value | Interpretation |
---|---|---|
Small Effect | 0.2 | Minimal practical significance |
Moderate Effect | 0.5 | Noticeable difference |
Large Effect | 0.8 | Substantial practical importance |
The formula for Cohen’s d in a one-sample t-test is \(d = \frac{m – \mu}{s}\). Here, \(m\) is the sample mean, \(\mu\) is the population mean, and \(s\) is the standard deviation. 🧮
Statistical significance doesn’t always mean practical significance. A small p-value might show a statistically significant result. However, the effect size reveals the true impact of findings.
Researchers must consider both aspects for thorough data interpretation. This approach ensures a complete understanding of research results. 🚀
Real-World Applications and Examples
Statistical methods like the one-sample t-test formula offer valuable insights across many fields. They help researchers and professionals make informed decisions based on sample data. This tool turns raw numbers into meaningful conclusions.
The one-sample t-test formula compares sample means to expected population values. It’s crucial in fields that need precise measurement and statistical validation. This technique proves invaluable across various professional domains.
Engineering Quality Control
Engineers use the one-sample t-test to ensure product consistency in manufacturing. For example, when testing battery life, researchers can check if performance matches design specs. They can also validate manufacturing processes and spot production variations.
Scientific Research Applications
Scientists often use the one-sample t-test in their work. They compare experimental results to theoretical predictions and validate research hypotheses. This method helps them determine if their findings are statistically significant.
Business Decision Making
Business leaders apply the one-sample t-test formula in various ways. They assess customer satisfaction scores and evaluate performance metrics. This data-driven approach helps them make strategic decisions for their companies.
The one-sample t-test helps professionals in many industries make sense of data. It transforms raw numbers into actionable insights, guiding important decisions. 🚀
Conclusion
The one-sample t-test is a powerful statistical tool. It allows precise comparisons between sample means and population parameters. This technique helps researchers analyze data across various fields.
Statistical hypothesis testing offers robust methods for understanding variability and significance. The one-sample t-test provides a systematic approach to drawing conclusions from sample data. It’s useful in examining diverse topics like sleep habits and professional demographics.
Researchers must remember key assumptions for this method. Normal data distribution is crucial. Understanding degrees of freedom and interpreting p-values are also essential. The Sign Test is an alternative when standard assumptions don’t apply.
This knowledge helps transform raw data into actionable insights. You can now make informed decisions based on quantitative evidence. Keep exploring and practicing data analysis techniques. 📊🔬
FAQ
What is a one-sample t-test?
A one-sample t-test compares a sample mean to a known population mean. It helps researchers test hypotheses about populations. This method works well for small sample sizes and continuous data.
When should I use a one-sample t-test?
Use it to compare a sample mean to a known population mean. It’s best for small samples under 30. This test suits continuous data following a normal distribution.
It’s great for comparing product performance or evaluating new treatments.
What are the key assumptions for a one-sample t-test?
The data should be normally distributed and randomly selected. The sample size should be small, typically less than 30. There should be no significant outliers.
Lastly, the observations should be independent of each other.
What’s the difference between a one-tailed and two-tailed t-test?
A one-tailed t-test checks if the sample mean differs in one specific direction. A two-tailed t-test looks for differences in either direction.
How do I interpret the p-value in a one-sample t-test?
The p-value shows the probability of getting extreme results if the null hypothesis is true. A small p-value (≤ 0.05) suggests strong evidence against the null hypothesis.
What is the significance of the significance level in a t-test?
The significance level is a threshold for deciding statistical significance. It’s often set at 0.05. This represents the chance of rejecting a true null hypothesis.
A lower level means a more conservative approach to claiming significance.
How do I calculate degrees of freedom in a one-sample t-test?
Subtract 1 from the sample size to get degrees of freedom. For example, a sample of 25 has 24 degrees of freedom.
This value helps determine the critical t-value and interpret results.
Can I use a t-test with non-normally distributed data?
T-tests can handle minor deviations from normal distribution, especially with larger samples. For very non-normal data, try non-parametric tests like the Wilcoxon signed-rank test.
You can also use data transformation to approach normality.