Mastering the P-Value from Chi-Square: A Step-by-Step Guide
Staff Writer • April 4, 2025 • Analytics, Marketing
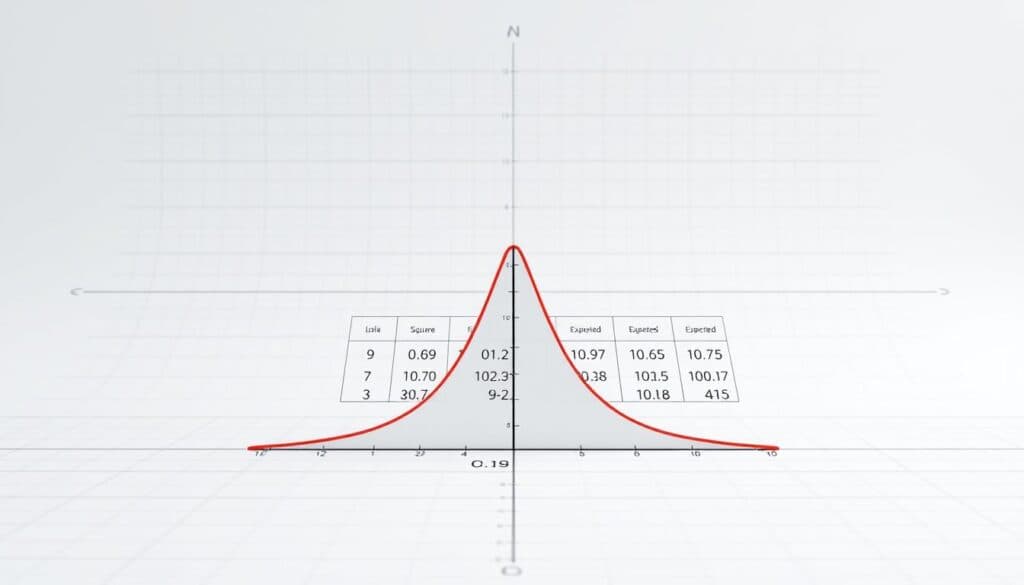
Statistical tests validate 95% of scientific research findings. The Chi-Square test analyzes categorical data and uncovers hidden relationships. Learning to find p-value from chi-square turns raw numbers into meaningful insights.
Statistical analysis can be simple and straightforward. This guide breaks down chi-square p-value calculation into easy steps. It’s perfect for researchers, business analysts, and data enthusiasts.
Chi-Square tests are used in many fields. They examine relationships between observed and expected frequencies. Professionals use this method to uncover patterns in categorical data for various studies.
Key Takeaways
- Chi-Square tests help analyze relationships in categorical data
- Understanding p-values is crucial for statistical interpretation
- The test works across various research and business contexts
- Statistical software can simplify chi-square calculations
- Proper interpretation requires understanding degrees of freedom
Understanding Chi-Square Test Fundamentals
The Chi-Square test is a powerful statistical tool. It helps uncover relationships between categorical variables. This test reveals patterns in data that might not be immediately visible.
The p value chi square test has core principles worth exploring. It compares observed data with expected frequencies. This makes it crucial for research and data analysis.
What is a Chi-Square Test?
A Chi-Square test analyzes categorical data to find significant relationships. It compares observed and expected frequencies. This allows researchers to draw meaningful conclusions about statistical patterns in their data.
Types of Chi-Square Tests
- Chi-Square Test of Independence: Examines relationships between two categorical variables
- Chi-Square Goodness-of-Fit Test: Determines if observed data matches expected distribution
The Basic Chi-Square Formula
Understanding the p value in chi square test requires knowing its basic calculation. The formula compares observed frequencies with expected ones across different categories.
Test Type | Primary Purpose | Application |
---|---|---|
Independence Test | Analyze variable relationships | Market research, social sciences |
Goodness-of-Fit Test | Compare observed to expected distributions | Quality control, population studies |
Chi-Square tests help make statistical inferences about categorical data. They provide a rigorous method for understanding complex relationships. This test offers valuable insights into data patterns for various fields.
How to Find P Value from Chi Square
Finding p value from chi square is simpler than you might expect. Let’s break down the process into easy steps. You’ll soon be able to interpret your data with confidence.
Calculating the Chi-Square Statistic
The chi-square test starts with calculating the chi-square statistic. The formula is: χ² = Σ [(O – E)² / E].
O stands for observed values, while E represents expected values. Σ means you’ll add up calculations for all data points.
- O represents the observed values
- E represents the expected values
- Σ indicates you’ll sum up the calculations for all data points
Determining Degrees of Freedom
Degrees of freedom are vital in statistical analysis. For a contingency table, use this formula: df = (r-1)(c-1).
- r = number of rows
- c = number of columns
Converting Chi-Square to P-Value
After finding your chi-square statistic, you’ll need to convert it to a p-value. Here’s a quick guide for significance levels:
Chi-Square Value (Χ²) | P-Value | Significance Level |
---|---|---|
3.8416 | 0.05 | 95% Confidence |
5.9915 | 0.05 | 99% Confidence |
9.5493 | 0.01 | 99% Confidence |
A p-value below 0.05 usually shows statistically significant results. Use statistical software or online calculators to make this process easier. They can help reduce errors too.
Statistical Significance and Hypothesis Testing
Statistical significance is key in chi-square test calculations. Researchers use methods to test claims against observed data. This process is called hypothesis testing. 🔍
Hypothesis testing has two main parts: the null hypothesis (H0) and the alternative hypothesis (Ha). The null hypothesis assumes no significant relationship between variables. 📊
- Null Hypothesis (H0): No significant difference or relationship
- Alternative Hypothesis (Ha): Significant difference or relationship exists
In chi-square tests, a 0.05 (5%) significance level is common. If the p-value is below 0.05, you can reject the null hypothesis. This gives you 95% confidence. 🧠
Significance Level | Interpretation |
---|---|
0.05 (5%) | Standard threshold for most research |
0.01 (1%) | Stricter evidence requirement |
0.001 (0.1%) | Extremely high confidence level |
Errors are important to understand in hypothesis testing. Type I errors reject a true null hypothesis. Type II errors fail to reject a false null hypothesis. 🚨
Careful analysis helps minimize these risks. Mastering these concepts improves your data interpretation skills. You’ll make better data-driven decisions with more confidence! 🎯
Interpreting Chi-Square Test Results
Statistical analysis demands attention to detail and understanding complex data. Interpreting p value in chi square tests is vital for making statistical inferences. This skill helps researchers draw meaningful conclusions from their data.
Chi-square p value calculation offers powerful insights into research findings. Let’s explore the key aspects of interpreting these results!
Understanding P-Value Thresholds
Statistical significance relies on specific p-value benchmarks:
- P-value
- P-value
- Values above 0.05 suggest non-significant results
Effect Size Measurements
Researchers use additional metrics to assess relationship strength:
- Cramer’s V: Measures association strength
- Phi Coefficient: Determines direction of relationship
- Adjusted Residuals: Identify specific cell contributions
Making Statistical Inferences
Successful data interpretation goes beyond number crunching. Consider these approaches when calculating chi-square p values:
- Examine both statistical significance and practical relevance
- Compare observed vs. expected frequencies
- Context matters more than raw numbers
A low p-value doesn’t always mean a groundbreaking discovery. Careful analysis and expertise are crucial for turning results into actionable insights!
Practical Applications in Data Analysis
Chi-Square tests have transformed data analysis across industries, offering powerful insights into complex relationships. Professionals use these tests to uncover hidden patterns and make data-driven decisions. The chi square test significance level is crucial in this process.
Here are some practical applications where calculating significance level for chi square test is vital:
- Healthcare Research: Investigating disease correlations
- Market Research: Analyzing consumer behavior trends
- Social Sciences: Examining demographic interactions
- Quality Control: Detecting manufacturing variations
Medical researchers use Chi-Square tests to find connections between variables. For example, a study might explore links between smoking and lung cancer in specific groups. They often use the chi-square statistical method for this purpose.
Industry | Application | Key Insight |
---|---|---|
Healthcare | Disease Risk Assessment | Identifying statistical correlations |
Marketing | Consumer Preference Analysis | Understanding purchasing behaviors |
Education | Student Performance Evaluation | Detecting significant learning patterns |
Market researchers use Chi-Square tests to understand customer preferences through survey data. They evaluate customer experience metrics to make strategic decisions for business growth. This approach drives innovation and improves products.
Mastering Chi-Square tests can enhance your analytical skills significantly. These powerful statistical techniques can reveal hidden patterns in your data. Start exploring them today to gain deeper insights!
Conclusion
Finding p-value from chi-square tests is crucial in statistical analysis. It’s a powerful tool for examining relationships between categorical variables. This method helps make informed decisions based on evidence. 🔍
P-value chi-square tests reveal significant patterns in data. They transform raw numbers into meaningful insights. This helps organizations and researchers validate hypotheses with confidence. 🚀
Mastering chi-square tests takes practice and careful interpretation. Each calculation develops deeper analytical skills. It uncovers nuanced relationships within complex datasets. Statistical understanding goes beyond numbers. 💡
Your statistical analysis journey is just beginning. Refining these skills unlocks powerful data-driven decision-making strategies. This applies to business, academic, and research fields.
Keep exploring and learning. Let curiosity drive your statistical adventures! 🌟
FAQ
What is a Chi-Square test?
A Chi-Square test analyzes categorical data to find relationships between variables. It’s used in market research, social sciences, and healthcare. This tool helps understand patterns in discrete data sets.
How do I calculate the p-value in a Chi-Square test?
To calculate the p-value, first find the Chi-Square statistic. Then, determine the degrees of freedom. Use a Chi-Square distribution table or software to find the p-value.
Finally, compare the p-value to your chosen significance level, usually 0.05.
What does a p-value tell me in a Chi-Square test?
The p-value shows the likelihood of getting extreme results if the null hypothesis is true. A low p-value (≤ 0.05) suggests a significant relationship between variables.
What are the two main types of Chi-Square tests?
The two main types are the Chi-Square Test of Independence and the Chi-Square Goodness-of-Fit Test. The first checks relationships between two categorical variables.
The second compares observed and expected frequencies in a single categorical variable.
How do I interpret the significance level in a Chi-Square test?
The standard significance level is 0.05. If p-value ≤ 0.05, reject the null hypothesis. If p-value > 0.05, fail to reject the null hypothesis.
Remember, statistical significance doesn’t always mean practical significance in real-world situations.
What factors affect the Chi-Square test results?
Several factors impact Chi-Square test results. These include sample size, number of categories, and degrees of freedom. Data collection accuracy and test assumptions also play a role.
Can I use Chi-Square tests for all types of data?
Chi-Square tests are designed for categorical data, not continuous variables. They work best with frequency data and discrete outcomes. The data should be countable and fit into exclusive categories.
How do I choose the right significance level?
While 0.05 is standard, the significance level can vary. It depends on the research field and potential error consequences. Some fields may use 0.01 or 0.10 based on context and risk tolerance.